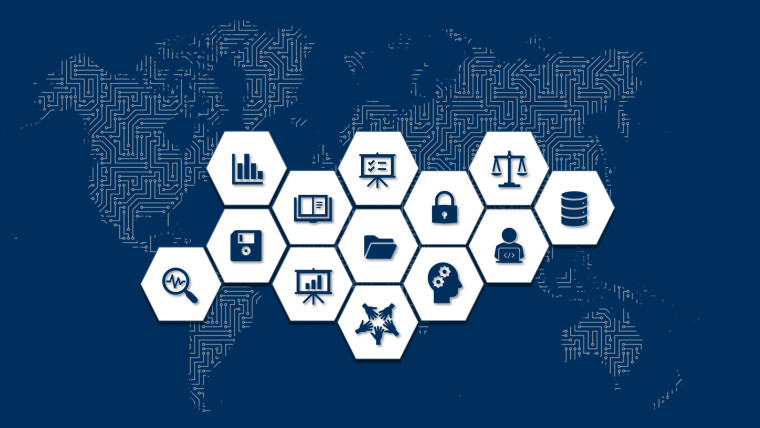
Overlap between Data Literacy and related competencies
Picture: Volker SchwartzeAs a result of the ongoing digitisation and datafication of all research fields, competencies regarding the handling of research data become more and more important. Thus, Data Literacy is not a specific competency for certain experts like Data Scientists or Data Stewards but a fundamental core competency in all fields of research. These competencies are not only important in science and are part of the so-called "Future Skills", a collection of skills that will become increasingly important regarding workplaces but also regarding the social participation in the next few years.
Data Literacy encompasses a great variety of individual competencies from related topics like statistics, digital skills and visualisation. In addition, also ethical and legal aspects as well as discipline-specific expertises are part of Data Literacy.
Due to the broad definition and the variety of competencies, Data Literacy goes way beyond the traditional borders of the individual research fields. Therefore, Data Literacy competencies are not or only partially implemented in most study programmes to date.
Data Literacy should not be understood as a synonym for Data Science. While Data Science represents an independent research field and includes mainly technical competencies regarding Statistics and Computer Science, Data Literacy is a basic competency that is relevant for every person at a certain level of expertise.
The following overview is based on the Data Literacy competency matrix from Ridsdale et al. (2015)4, which is one of the most widely used competence frameworks to date. The 21 individual competencies from 5 knowledge areas are categorised into conceptual, basic and advanced competencies. Further descriptions and examples for the competencies are given.
-
Conceptual Framework
Introduction to Data (conceptual)
- Basic knowledge and understanding of data
- Understanding of relevance and applications of data
- Knowledge about different data types and formats
-
Data Discovery and Collection
Data Collection (basic)
- Identification of relevant data
- Collection of data using appropriate methods (e.g. observations, measurements, simulations)
- Search for and collection of existing data
- Example: use of search engines and databases
Evaluating and Ensuring Quality of Data and Sources (basic)
- Critical assessment of data sources for trustworthiness
- Critical evaluation of the quality of datasets (e.g. errors, completeness, relevance, representativity)
-
Data Management
Data Organisation (basic)
- Knowledge of basic data organisation methods and tools
- Assessment of data organisation requirements
- Example: meaningful naming of files, development of logical folder structures, structure of spreadsheets
Data Manipulation (basic)
- Assessment of methods to clean data
- Identification of outliers and anomalies
- Processing of data (e.g. filter, sort, merge)
Data Conversion (advanced)
- Knowledge of different data types and conversion methods
- Conversion of data from one format or file type to another
Metadata Creation and Use (advanced)
- Awareness for the importance of data documentation
- Creation of metadata descriptors
- Assignment of appropriate metadata descriptors to original data sets
- Application of relevant methods and standards
Data Curation, Security, and Re-Use (advanced)
- Assessment of data curation requirements (e.g. retention schedule, storage, accessibility, sharing requirements, etc.)
- Assessment of data security requirements (e.g. restricted access, protected drives, etc.)
Data Preservation (advanced)
- Assessment of requirements for preservation and application of appropriate methods / tools
-
Data Evaluation
Data Tools (conceptual)
- Knowledge of data analysis tools and techniques
- Selection of appropriate data analysis tool or technique
- Application of data analysis tools and techniques
Basic Data Analysis (basic)
- Development of analysis plans
- Application of analysis methods and tools
- Implementation of exploratory analyses
- Evaluation of results of analysis and comparison to results of other analyses
Data Interpretation (Understanding Data) (basic)
- Ability to read and understand charts, tables and graphs
- Identification of key messages and integration with other important information
- Identification of discrepancies within the data
Identifying Problems Using Data (basic)
- Use of data to identify problems in practical situations (e.g. workplace efficiency)
- Use of data to identify higher level problems (e.g. politics, environment, scientific experimentation, marketing, economics, etc.)
Data Visualisation (basic)
- Creation of meaningful tables to organise and visually present data
- Creation of meaningful graphical representations of data
- Evaluation of the effectiveness of graphical representations
- Critical assessment of graphical representations in terms of accuracy and misrepresentation of data
Presenting Data (basic)
- Assessment of the desired outcome(s) for the data presentation
- Assessment of audience needs and familiarity with the subject(s)
- Utilisation of meaningful tables and visualisations
- Clear and coherent presentation of arguments and/or outcomes
Data Driven Decisions (basic)
- Prioritisation of information garnered from data
- Conversion of data into actionable information
- Weighing the merit and impacts of possible solutions / decisions
- Implementation of decisions / solutions
-
Data Application
Critical Thinking (conceptual)
- Awareness of high-level / complex issues and challenges associated with data
- Critical thinking with respect to working with data
Data Culture (conceptual)
- Recognition of the importance of data
- Support of an environment that fosters critical use of data in education, research, and decision-making
Data Ethics (conceptual)
- Awareness of legal and ethical issues associated with data
- Application of and working with data in an ethical manner
Data Citation (basic)
- Knowledge of widely accepted data citation methods
- Creation of correct citations for secondary data sets
Data Sharing (basic)
- Assessment of methods and platforms for data sharing
- Sharing of data in a legal and ethical manner
Evaluation of Decisions Based on Data (advanced)
- Collection of follow-up data to assess effectiveness of decisions or solutions based on data
- Analysis of follow-up data and comparison of the results with other findings
- Evaluation of decisions or solutions based on data
1 Duden (online), Stand: 14.01.2021
2 Jakob Voß (2013) Was sind eigentlich Daten? LIBREAS. Library Ideas, 23: 4-11, DOI: http://dx.doi.org/10.18452/9038.
3 Schüller K., Busch P., Hindinger C. (2019) Future Skills: Ein Framework für Data Literacy. Zenodo. DOI: 10.5281/zenodo.3349865
4 Ridsdale C., Rothwell J., Smit M., Ali-Hassan H., Bliemel M., Irvine D., Kelley D., Matwin S., Wuetherick B. (2015) Strategies and Best Practices for Data Literacy Education Knowledge Synthesis Report. DOI: 10.13140/RG.2.1.1922.5044